Ryan Magee
Research Scientist | Caltech
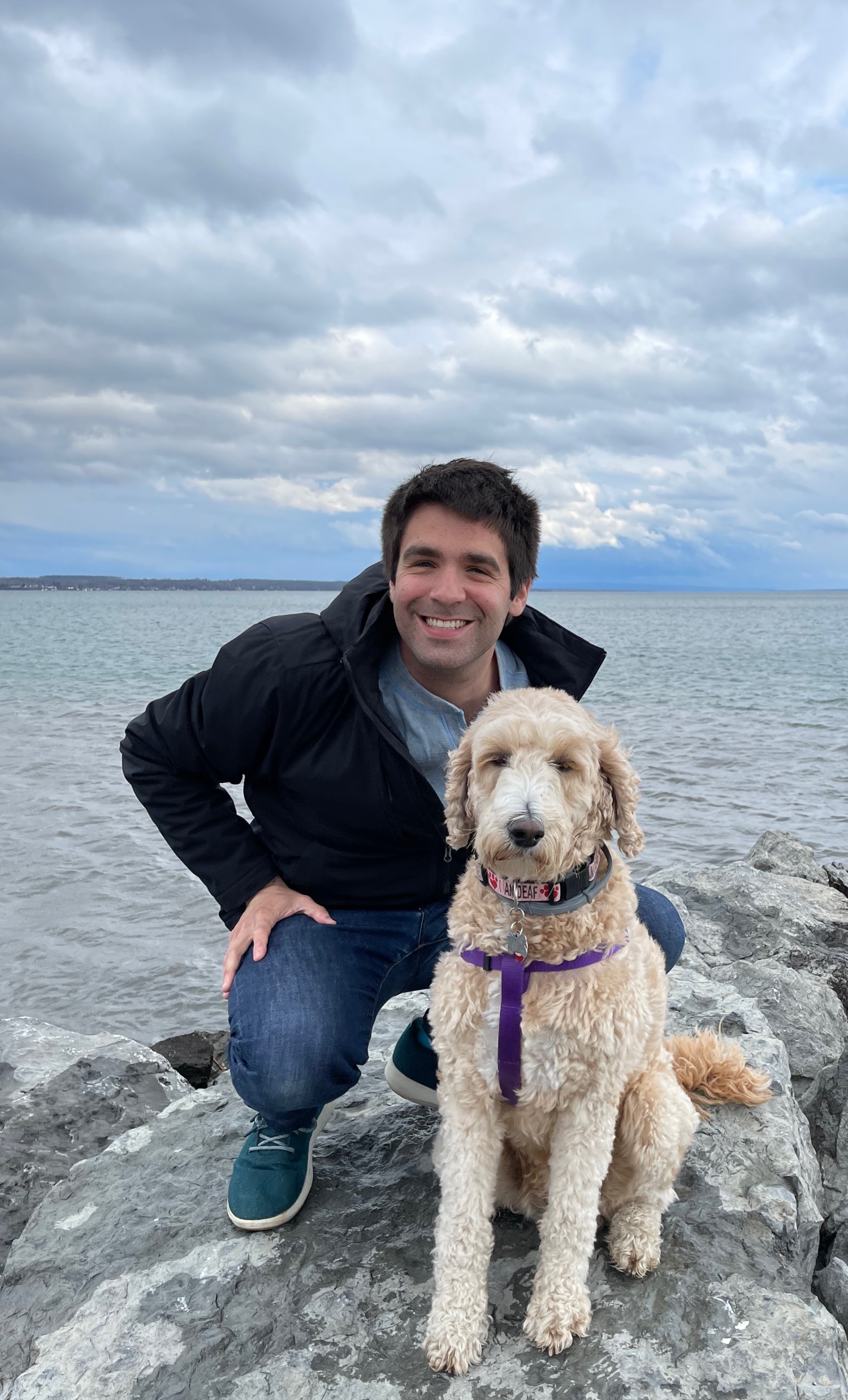
Research Scientist | Caltech
I am a Research Scientist at Caltech working within the data analysis group of the LIGO-Lab.
Gravitational-waves have given us a new window into extreme physical phenomena
across our Universe. My research focuses on the application of novel computing
paradigms to open questions in gravitational-wave astrophysics and cosmology. I
am particularly interested in facilitating additional multi-messenger
discoveries, using gravitational waves to probe fundamental physics, and exploring the
use of machine learning techniques in data-driven astrophysics.
For a more complete description of my work, please see my CV.
Please feel free to use images and animations from this page in talks with
appropriate credit. I produced all content on this page. Unless explicitly
noted, no real gravitational-wave data have been used. No proprietary data have
been used in any situation.